What is the purpose of EyeQ Trainer?
“The launch of EyeQ marks the arrival of one of the biggest premium video platforms in digital media,” ViacomCBS COO of Ad Revenue John Halley said in a press release. Unlock the hidden details and make photos tack sharp with our incredible sharpening technology. Automatically remove noise without blurring or losing details. Perfectly Clear automatically reduces noise 2-3 stops (unlike other tools that require multiple manual sliders). ISO 1600 effectively is reduced to ISO 200-400.
The purpose of the EyeQ Trainer is to improve vision skills where dysfunction was identified by a Doctor with support from the Functional Vision EyeQ test.
What areas of the eye and brain does EyeQ Trainer claim to rehabilitate?
- Trains all 12 muscles of the eyes
- Rehabilitates all 6 movement systems of the eyes
- Promotes positive plasticity in those neural substrates that support claims 1 and 2
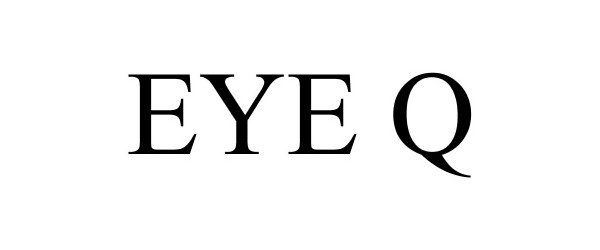
Why does EyeQ Trainer work?
EyeQ Trainer is based on well known and understood mapping of visual and brain circuits outlined by Dr. John Leigh and Dr. David Zee in The Neurology of Eye Movements. These circuits are activated when a person moves their eyes in certain directions using specific eye movements.
The six eye movement systems can be functionally divided into two categories according to Wong (2008). The first category are those eye movements that hold the image of a target steady on the retina. The second category of eye movements direct the fovea onto an object of interest.
Category 1: eye movements that hold the image of a target steady on the retina.
- The fixationsystem: holds the image of a stationary object on the fovea when the head is still.
- The vestibular system (also known as the Vestibular-Ocular Reflex[VOR]): holds the image of a target steady on the retina during brief head movements.
- The optokinetic system: holds the image of a target steady on the retina during sustained head movement.
Category 2: eye movements that direct the fovea onto an object of interest.
- The saccadic system: brings the image of an object of interest rapidly onto the fovea
- The smooth pursuit system: holds the image of a small, moving target on the fovea
- The vergence system: moves the eyes in an opposite direction (converging or diverging) so that images of a single object are held simultaneously on both fovea.

What proof is there that it works?
Validity by design also considered “face validity” or “priori validity” is concerned with whether the EyeQ Trainer measures or “trains” what is being claimed (above). The above musculature and circuitry is validated by medical research (e.g. Sharpe & Wong, 2005; Standring, 2016). Anatomy and physiology are further validated by Adler & Milhorat (2002) in over 100 human autopsy cases and by medical research such as magnetic resonance imaging (e.g. Alkan, Sigirci, Ozveren et al, 2004).
The EyeQ Trainer activates the eye movement muscles and circuitry through various training exercises. These exercises are digitized representations of standard clinical care and in-office therapies employed by functional neurologists and optometrists (Press, 2008). Furthermore, the American Optometric Association (AOA) paper titled Fact Sheets on Optometric Vision Therapy states: Optometric vision therapy (on which EyeQ Trainer is based) has been shown to be an effective treatment modality for many types of problems affecting the vision system. Furthermore, here are dozens of before and after case-studies available that demonstrate effectiveness of EyeQ Trainer exercises.
References
Adler, D.E. & Milhorat, T.H. (2002). The tentorial notch: Anatomical variation, morphometric analysis, and classification in 100 human autopsy cases. Journal of Neurosurgery, 96: 1103-1112.
Alkan, A. Sigirci, A., Ozveren, M.F. et al. (2004). The cisternal segment of the abducens nerve in man: three-dimensional MR imaging. European Journal of Radiology, 51: 218-222.
Leigh, R.J. & Zee, D.S. (2015). The Neurology of Eye Movements. 5th Ed. Oxford University Press. New York, NY.
Press, L.J. (2008). Applied Concepts in Vision Therapy. St Louis Mosby, St. Louis, MI.
Standring, S. (2016). Greys Anatomy: The Anatomical Basis of Clinical Practice. Elsevier. New York, NY.
Sharp, J.A., Wong, A.M. (2005). Anatomy and physiology of ocular systems. In Millar, N.R., Newman, N.J., Bi-Lippincott Williams a& Wilkins, 809-885.
Wong, A.M.F. (2008) Eye Movements Disorders. Oxford University Press. New York, NY. Pp. 15.
Mobileye is the global leader in the development of vision technology for Advanced Driver Assistance Systems (ADAS) and autonomous driving.
We have over 1,700 employees continuing our two-decade tradition of developing state-of-the-art technologies in support of automotive safety and autonomous driving solutions.
Mobileye is a Tier 2 automotive supplier working with all major Tier 1 suppliers, covering the vast majority of the automotive market (programs with over 25 OEMs). These OEMs choose Mobileye, for its advanced technology, innovation culture, and agility. As a direct result, the robustness and performance of our technology have been battle-tested over millions of driving miles as part of the stringent validation processes of safety-critical automotive products.
From the beginning, Mobileye has developed hardware and software in-house. This has facilitated the strategic advantage of responsive and short development cycles of highly interdependent hardware, software and algorithmic stacks. This interdependence is key to producing high-performance and low power consumption products.
Mobileye’s system-on-chip (SoC) – the EyeQ® family – provides the processing power to support a comprehensive suite of ADAS functions based on a single camera sensor. In its fourth and fifth generations, EyeQ® will further support semi and fully autonomous driving, having the bandwidth/throughput to stream and process the full set of surround cameras, radars and LiDARs.
ADAS
Advanced Driver Assistance Systems (ADAS) systems range on the spectrum of passive/active.
A passive system alerts the driver of a potentially dangerous situation so that the driver can take action to correct it. For example, Lane Departure Warning (LDW) alerts the driver of unintended/unindicated lane departure; Forward Collision Warning (FCW) indicates that under the current dynamics relative to the vehicle ahead, a collision is imminent. The driver then needs to brake in order to avoid the collision.
In contrast, active safety systems take action. Automatic Emergency Braking (AEB) identifies the imminent collision and brakes without any driver intervention. Other examples of active functions are Adaptive Cruise Control (ACC), Lane Keeping Assist (LKA), Lane Centering (LC), and Traffic Jam Assist (TJA).
ACC automatically adjusts the host vehicle speed from its pre-set value (as in standard cruise control) in case of a slower vehicle in its path. LKA and LC automatically steer the vehicle to stay within the lane boundaries. TJA is a combination of both ACC and LC under traffic jam conditions. It is these automated features which comprise the building blocks of semi/fully autonomous driving.
Mobileye supports a comprehensive suite of ADAS functions – AEB, LDW, FCW, LKA, LC, TJA, Traffic Sign Recognition (TSR), and Intelligent High-beam Control (IHC) – using a single camera mounted on the windshield, processed by a single EyeQ® chip.
In addition to the delivery of these ADAS products through integration with automotive OEMs, Mobileye offers an aftermarket warning-only system that can be retrofitted onto any existing vehicle. The Mobileye aftermarket product offers numerous life-saving warnings in a single bundle, protecting the driver against the dangers of distraction and fatigue. Find more on the aftermarket product here.
Computer Vision
From the outset, Mobileye’s philosophy has been that if a human can drive a car based on vision alone – so can a computer. Meaning, cameras are critical to allow an automated system to reach human-level perception/actuation: there is an abundant amount of information (explicit and implicit) that only camera sensors with full 360 degree coverage can extract, making it the backbone of any automotive sensing suite.
It is this early recognition – nearly two decades ago – of the camera sensor superiority and investment in its development, that led Mobileye to become the global leader in computer vision for automotive.
Mobileye’s approach to the development of camera capabilities has always been to first produce optimal, self-contained camera-only products, demonstrated and validated to serve all functional needs. As a showcase, our demonstration vehicle drives autonomously from Jerusalem to Tel Aviv and back relying on camera sensors alone, while series-production autonomous vehicles fuse-in additional sensors for delivering a robust, redundant solution based on multiple modalities (mainly radar and LiDAR).
From ADAS to Autonomous
The road from ADAS to full autonomy depends on mastering three technological pillars:
Eyeq Infinite Mind
- Sensing: robust and comprehensive human-level perception of the vehicle’s environment, and all actionable cues within it.
- Mapping: as a means of path awareness and foresight, providing redundancy to the camera’s real-time path sensing.
- Driving Policy: the decision-making layer which, given the Environmental Model – assesses threats, plans maneuvers, and negotiates the multi-agent game of traffic.
Only the combination of these three pillars will make fully autonomous driving a reality.
The Sensing Challenge
Perception of a comprehensive Environmental Model breaks down into four main challenges:
- Freespace: determining the drivable area and its delimiters
- Driving Paths: the geometry of the routes within the drivable area
- Moving Objects: all road users within the drivable area or path
- Scene Semantics: the vast vocabulary of visual cues (explicit and implicit) such as traffic lights and their color, traffic signs, turn indicators, pedestrian gaze direction, on-road markings, etc.
The Mapping Challenge
The need for a map to enable fully autonomous driving stems from the fact that functional safety standards require back-up sensors – “redundancy” – for all elements of the chain – from sensing to actuation. Within sensing, this applies to all four elements mentioned above.
While other sensors such as radar and LiDAR may provide redundancy for object detection – the camera is the only real-time sensor for driving path geometry and other static scene semantics (such as traffic signs, on-road markings, etc.). Therefore, for path sensing and foresight purposes, only a highly accurate map can serve as the source of redundancy.
In order for the map to be a reliable source of redundancy, it must be updated with an ultra-high refresh rate to secure its low Time to Reflect Reality (TTRR) qualities.
To address this challenge, Mobileye is paving the way for harnessing the power of the crowd: exploiting the proliferation of camera-based ADAS systems to build and maintain in near-real-time an accurate map of the environment.
Mobileye’s Road Experience Management (REMTM) is an end-to-end mapping and localization engine for full autonomy. The solution is comprised of three layers: harvesting agents (any camera-equipped vehicle), map aggregating server (cloud), and map-consuming agents (autonomous vehicle).
The harvesting agents collect and transmit data about the driving path’s geometry and stationary landmarks around it. Mobileye’s real-time geometrical and semantic analysis, implemented in the harvesting agent, allows it to compress the map-relevant information – facilitating very small communication bandwidth (less than 10KB/km on average).
The relevant data is packed into small capsules called Road Segment Data (RSD) and sent to the cloud. The cloud server aggregates and reconciles the continuous stream of RSDs – a process resulting in a highly accurate and low TTRR map, called “Roadbook.”
The last link in the mapping chain is localization: in order for any map to be used by an autonomous vehicle, the vehicle must be able to localize itself within it. Mobileye software running within the map-consuming agent (the autonomous vehicle) automatically localizes the vehicle within the Roadbook by real-time detection of all landmarks stored in it.
Further, REMTM provides the technical and commercial conduit for cross-industry information sharing. REMTM is designed to allow different OEMs to take part in the construction of this AD-critical asset (Roadbook) while receiving adequate and proportionate compensation for their RSD contributions.
Driving Policy
Where sensing detects the present, driving policy plans for the future. Human drivers plan ahead by negotiating with other road users mainly using motion cues – the “desires” of giving-way and taking-way are communicated to other vehicles and pedestrians through steering, braking and acceleration. These “negotiations” take place all the time and are fairly complicated – which is one of the main reasons human drivers take many driving lessons and need an extended period of training until we master the art of driving. Moreover, the “norms” of negotiation vary from region to region as the code of driving in Massachusetts, for example, is quite different from that of California, even though the rules are identical.
The challenge behind making a robotic system control a car is that for the foreseeable future the “other” road users are likely to be human-driven, therefore in order not to obstruct traffic, the robotic car should display human negotiation skills but at the same time guarantee functional safety. In other words, we would like the robotic car to drive safely, yet conform to the driving norms of the region. Mobileye believes that the driving environment is too complex for hand-crafted rule-based decision making. Instead we adopt the use of machine learning to “learn” the decision making process through exposure to data.

Eyeq Monitoring
Mobileye’s approach to this challenge is to employ what is called reinforcement learning algorithms trained through deep networks. This requires training the vehicle system through increasingly complex simulations by rewarding good behavior and punishing bad behavior. Our proprietary reinforcement learning algorithms add human-like driving skills to the vehicle system, in addition to the super-human sight and reaction times that our sensing and computing platforms provide. It also allows the system to negotiate with other human-driven vehicles in complex situations. Knowing how to do this well is one of the most critical enablers for safe autonomous driving.
Eyeq Speed Reading
For more details on the challenges of using reinforcement learning for driving policy and Mobileye’s approach to the problem, please see: S. Shalev-Shwartz, S. Shammah and A. Shashua. Safe, Multi-Agent, Reinforcement Learning for Autonomous Driving. NIPS Workshop on Learning, Inference and Control of Multi-Agent Systems: Dec., 2016.